
[ad_1]
In a latest examine revealed in npj Digital Medicine, researchers developed diagnostic reasoning prompts to analyze whether or not giant language fashions (LLMs) may simulate diagnostic scientific causes.
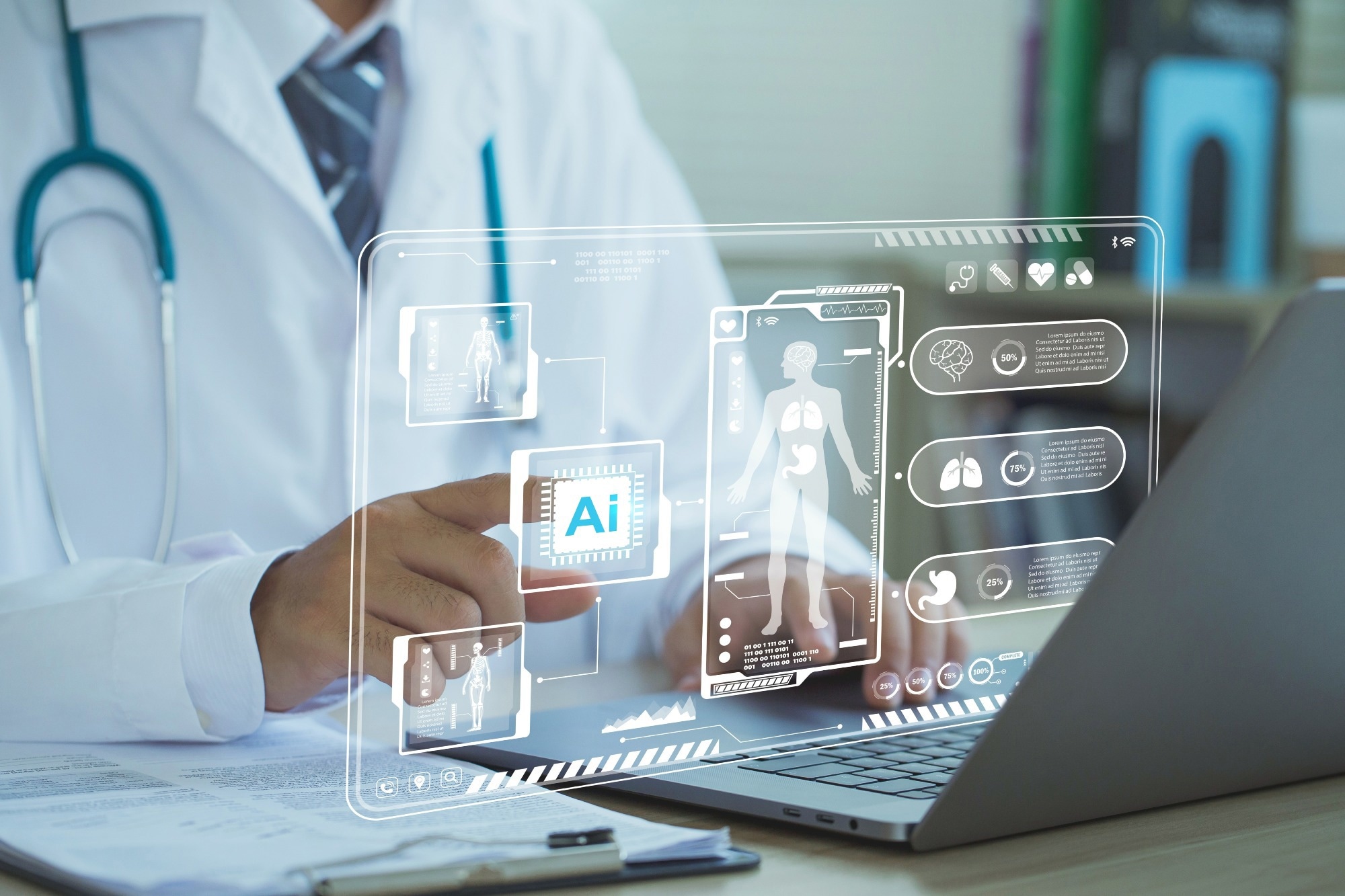
LLMs, synthetic intelligence-based methods educated utilizing huge quantities of textual content information, are recognized for human-simulating performances in duties like writing scientific notes and passing medical exams. However, understanding their scientific diagnostic reasoning skills is essential for his or her integration into scientific care.
Recent research have targeting open-ended-type scientific questions, indicating that progressive large-language fashions, like GPT-4, have the potential to determine advanced sufferers. Prompt engineering has begun to beat this concern, as LLM efficiency varies based mostly on the kind of prompts and questions.
About the examine
In the current examine, researchers assessed diagnostic reasoning by GPT-3.5 and GPT-4 for open-ended-type scientific questions, hypothesizing that GPT fashions may outperform typical chain-of-thought (CoT) prompting with diagnostic reasoning prompts.
The crew used the revised MedQA United States Medical Licensing Exam (USMLE) dataset and the New England Journal of Medicine (NEJM) case collection to check typical chain-of-thought prompting with varied diagnostic logic prompts modeled after the cognitive procedures of forming differential analysis, analytical reasoning, Bayesian inferences, and intuitive reasoning.
They investigated whether or not large-language fashions can mimic scientific reasoning expertise utilizing specialised prompts, combining scientific experience with superior prompting methods.
The crew used immediate engineering to generate prompts for diagnostic reasoning, changing questions into free responses by eliminating multiple-choice alternatives. They included solely step II and step III questions from the USMLE dataset and people evaluating affected person analysis.
Each spherical of immediate engineering concerned GPT-3.5 accuracy analysis utilizing the MEDQA coaching set. The coaching and testing units, which contained 95 and 518 questions, respectively, have been reserved for evaluation.
The researchers additionally evaluated GPT-4 efficiency on 310 instances lately revealed within the NEJM journal. They excluded 10 that didn’t have definitive closing diagnoses or surpassed the utmost context size for GPT-4. They in contrast typical CoT prompting with the best-performing scientific diagnostic reasoning CoT prompts (reasoning for differential analysis) on the MedQA dataset.
Every immediate consisted of two exemplifying questions with rationales utilizing goal reasoning methods or few-shot studying. The examine analysis used free-response questions from the USMLE and NEJM case report collection to facilitate rigorous comparability between prompting methods.
Physician authors, attending physicians, and an inside drugs resident evaluated language mannequin responses, with every query assessed by two blinded physicians. A 3rd researcher resolved the disagreements. Physicians verified the accuracy of solutions utilizing software program when wanted.
Results
The examine reveals that GPT-4 prompts may mimic the scientific reasoning of clinicians with out compromising diagnostic accuracy, which is essential to assessing the accuracy of LLM responses, thereby enhancing their trustworthiness for affected person care. The strategy might help overcome the black field limitations of LLMs, bringing them nearer to secure and efficient use in drugs.
GPT-3.5 precisely responded to 46% of evaluation questions by customary CoT prompting and 31% by zero-shot-type non-chain-of-thought prompting. Of prompts related to scientific diagnostic reasoning, GPT-3.5 carried out one of the best with intuitive-type reasonings (48% versus 46%).
Compared to basic chain-of-thought, GPT-3.5 carried out considerably inferiorly with analytical reasoning prompts (40%) and people for growing differential diagnoses (38%), whereas Bayesian inferences fell wanting significance (42%). The crew noticed an inter-rater consensus of 97% for MedQA information GPT-3.5 evaluations.
The GPT-4 API returned errors for 20 check questions, limiting the dimensions of the check dataset to 498. GPT-4 displayed extra accuracy than GPT-3.5. GPT-4 confirmed 76%, 77%, 78%, 78%, and 72% accuracies with classical chain-of-thought, intuitive-type reasoning, differential diagnostic reasoning, analytical reasoning prompts, and Bayesian inferences, respectively. The inter-rater consensus was 99% for GPT-4 MedQA evaluations.
Regarding the NEJM dataset, GPT-4 scored 38% accuracy with typical CoT versus 34% with that for formulating differential analysis (a 4.2% distinction). The inter-rater consensus for the GPT-4 NEJM evaluation was 97%. GPT-4 responses and rationales for the whole NEJM dataset. Prompts selling step-by-step reasoning and specializing in a single diagnostic reasoning technique carried out higher than these combining a number of methods.
Overall, the examine findings confirmed that GPT-3.5 and GPT-4 have improved reasoning skills however not accuracy. GPT-4 carried out equally with typical and intuitive-type reasoning chain-of-thought prompts however worse with analytical and differential analysis prompts. Bayesian inferences and chain-of-thought prompting additionally confirmed worse efficiency in comparison with classical CoT.
The authors suggest three explanations for the distinction: the reasoning mechanisms of GPT-4 could possibly be integrally completely different from these of human suppliers; it may clarify post-hoc diagnostic evaluations in desired reasoning codecs; or it may attain most precision with the supplied vignette information.
[adinserter block=”4″]
[ad_2]
Source link