
[ad_1]
A drug screen using machine learning has identified hundreds of potential drugs that could be used to treat COVID-19, researchers say.
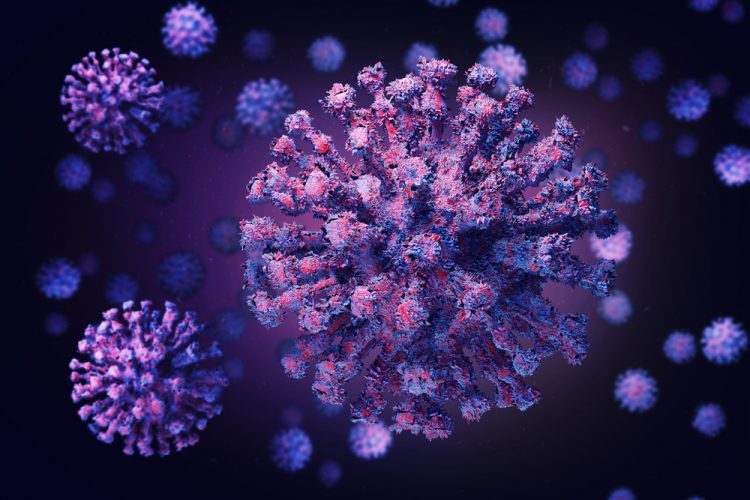
Researchers have used machine learning to identify hundreds of new potential drugs that could help treat COVID-19, the disease caused by SARS-CoV-2. The study was conducted at the University of California, Riverside, US.
“There is an urgent need to identify effective drugs that treat or prevent COVID-19,” said Professor Anandasankar Ray, who led the research. “We have developed a drug discovery pipeline that identified several candidates… Existing US Food and Drug Administration (FDA)-approved drugs that target one or more human proteins important for viral entry and replication are currently high priority for repurposing as new COVID-19 drugs. The demand is high for additional drugs or small molecules that can interfere with both entry and replication of SARS-CoV-2 in the body. Our drug discovery pipeline can help.”
Joel Kowalewski, a graduate student in Ray’s lab, used small numbers of previously known ligands for 65 human proteins that are known to interact with SARS-CoV-2 proteins. He generated machine learning models for each of the human proteins.
“These models are trained to identify new small molecule inhibitors and activators – the ligands – simply from their three-dimensional (3D) structures,” Kowalewski said.
Ray and Kowalewski used their models to screen more than 10 million commercially available small molecules from a database comprised of 200 million chemicals and identified the best-in-class hits for the 65 human proteins that interact with SARS-CoV-2 proteins. They were thus able to create a database of chemicals whose structures were predicted as interactors of the 65 protein targets.
The researchers then identified compounds among the hits that are already FDA approved. They also used the machine learning models to compute toxicity, which helped them reject potentially toxic candidates. This helped them prioritise the chemicals that were predicted to interact with SARS-CoV-2 targets. Their method allowed them to not only identify the highest scoring candidates with significant activity against a single human protein target, but also find a few chemicals that were predicted to inhibit two or more human protein targets.
“Machine learning approaches like ours can play a role in anticipating the evolving treatment landscape by providing researchers with additional possibilities for further study. While the approach crucially depends on experimental data, virtual screening may help researchers ask new questions or find new insight,” Kowalewski said.
Ray and Kowalewski also argue that their computational strategy for the initial screening of vast numbers of chemicals has an advantage over traditional cell-culture-dependent assays that are expensive and can take years to test.
The results are published in Heliyon.
[ad_2]
Source link